In this edition of SigView – A Quant’s Perspective, we have the pleasure of speaking with Frank Haeusler, Chief Investment Strategist at the Swiss investment firm, Vontobel. In his 20+ year career, Frank has been instrumental in building high performing research and portfolio management teams at banks, asset managers and family offices. Whilst trained as a quant, he has found his speciality in the intersection of ‘man and machine’.
We hope you enjoy the conversation.
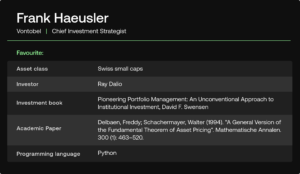
Tell us about your role at Vontobel.
In my role as Chief Investment Strategist at Vontobel, my team of approximately 30 investment professionals and I manage part of the firm’s multi-asset business. We are responsible for the full value chain ranging from research, selection, to portfolio construction and implementation (i.e. portfolio management). My key responsibility lies in determining the strategic asset allocation (SAA), tactical asset allocation (TAA) and the individual security selection within each asset class for the portfolios we run, and of course ensuring smooth PM operations. In addition to the expertise within my team, I can draw upon the vast resources of Vontobel’s global operations with 2,000+ employees in 26 worldwide locations.
What kind of investment strategies do you manage?
My team and I specialize in managing multi-asset class mandates for private wealth and digital clients, larger institutional investors and family offices. Our offering consists of both bespoke mandates and standardized solutions. We are not quant investors but we use quant tools for decision support.
How do you make use of quant models in your investment decision making process?
Quant models are an important part of our investment process and allow us to scale and speed up our research capacity. Examples of quant analytics we run are relative value, sentiment and macro models to compare the attractiveness of different asset classes. The underlying capital market assumptions to determine the SAA are ca. 90% based on quant models but the final portfolio allocation decision is always a result of a discretionary overlay. I am a strong believer in man and machine, not one or the other in isolation. When it comes to individual security selection in, for instance, the equity bucket of the portfolios we manage, we make use of various filtering techniques based mainly on fundamental data.
What is unique about your quant models?
The investment philosophy of my quant models is based on the three pillars of a top down macroview, behavioral anomalies, and valuation. I implement the same bottom-up style across all asset classes and apply a medium- to long-term model horizon. In terms of my approach to building quant models, I adhere to the old notion from Albert Einstein, ‘make everything as simple as possible, but not simpler.’ It is tempting – and not particularly difficult – to build complex models. You will always be able to find parameters that would have perfectly explained the past but from my experience they often have low predictive power going forward. That is why I try to avoid state-dependent models for example. But again, I want to reiterate that I believe quant models work best in combination with a human overlay and this is where I can find an additional edge based on my 20+ years as a fund and portfolio manager.
What are the key challenges you and your team face in your day-to-day operations to develop and maintain your quant models?
Given there never is a lack of research and trading ideas, the key for me is to prioritize what to work on and make sure the research process is as efficient as possible. This means that I need to ensure that I have the underlying quant infrastructure to allow us to focus on our core competencies of generating alpha for our investors. But, given there is always a human who decides in the end, it is not always easy to allocate enough resources to developing and running the quant models that support our investment decision making process. In addition, I want to mention that the time and cost it takes to source high quality data and to make it operationally-ready is often underestimated.
Given the current uncertain market environment, are there any specific quant models and datasets that you currently find particularly relevant? And, what are you looking to add to your current set-up?
Looking ahead to 2023, I expect the macroeconomic theme to continue to be the key narrative in the financial markets. However, fundamental data will again play an increasingly important role. Apart from constantly trying to expand the history of the datasets to incorporate as many market cycles as possible, I am currently researching how diffusion indices can help improve our forecasting capabilities for various macro variables and beyond.
Where do you see the most promising opportunities to generate alpha in the current market environment?
With the prolonged period of central banks’ zero interest rate policy coming to an end, I expect there to be a washout of ‘zombie’ companies with business models dependent on ‘free’ money. Thus, divergences in fundamentals will again be an important driver of asset prices and the increased alpha opportunity set will be beneficial for actively managed strategies over the coming years. This in particular holds true for unconstrained multi-asset strategies, as yields have started to normalize and fixed income again can play its role as a source of both diversification and returns.
In your interactions with larger institutional investors, how have attitudes to quant investing changed over time?
Quant has become a household name among investors. If you, for instance, analyze and invest in large investment universes you must apply some kind of quant analytics to be credible. The latest trend I have observed is that institutional investors increasingly want to take an in-depth look ‘under the hood’ to fully understand how quant models are built. The level of quant knowhow among investors has gone up significantly and I expect the trend to continue.
Disclaimer
SigTech is not responsible for, and expressly disclaims all liability for, damages of any kind arising out of use, reference to, or reliance on such information. While the speaker makes every effort to present accurate and reliable information, SigTech does not endorse, approve, or certify such information, nor does it guarantee the accuracy, completeness, efficacy, timeliness, or correct sequencing of such information. All presentations represent the opinions of the speaker and do not represent the position or the opinion of SigTech or its affiliates.