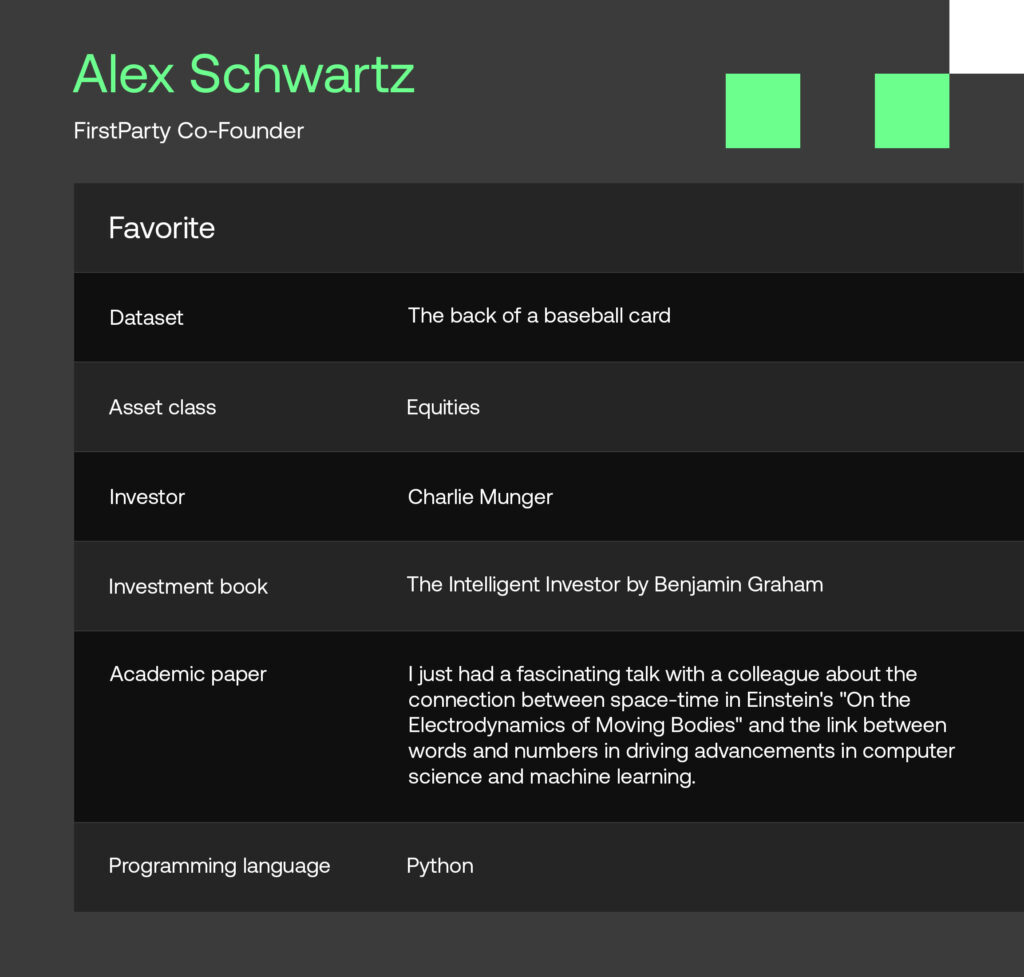
We are excited to feature Alex Schwartz and his valuable insights in this edition of SigView. Alex is Co-Founder of FirstParty, an organization helping companies extract value from their data. He discusses the biggest challenges in the industry today and the key problems users face in getting data operationally ready.
We hope you enjoy the conversation!
Tell us about your background.
I began my career at SAC Capital just prior to the Global Financial Crisis in 2008, beginning in operations before transitioning to a front office special projects role where I focused on the identification and trainability of skill in portfolio managers.
My work with portfolio managers exposed me to the increasing use of data in investing, and in 2013 I jumped at the opportunity to be the first hire and Head of Product at 7Park Data – a startup that wanted to distill the complexities of alternative datasets into actionable insights for finance and corporate audiences.
After a successful 5 year run at 7Park culminating in a sale to private equity firm Vista Equity Partners, I joined quantPort as a systematic portfolio manager. For 2 years I ran an experimental portfolio that leveraged alternative data exclusively to inform daily trading strategies.
With quantPort closing at the start of COVID, I was afforded some time to think about my next move. Having spent time in recent years as a data product builder and a data product consumer it was clear that some significant gaps in expertise and capabilities still existed for corporations sitting on data with massive potential. That theme, along with the longtime desire to run my own company, led me to co-found FirstParty in 2021.
Tell us about FirstParty.
FirstParty exists to help companies better understand and realize the value of their data assets. The alternative data industry is still in its infancy, and expertise in utilization and monetization are in short supply. This often results in organizational gridlock around the very same data initiatives that senior leaders have identified as critical to success.
We provide software, services, and people for our clients to overcome these barriers in order to achieve successful outcomes with their data initiatives. We’ve worked with clients ranging from PE-backed startups to Fortune 500 companies to identify their data’s value, apply best practices in data hygiene, and construct a data asset that is clean, stable, enriched, and staged for insight.
What do you view as the current biggest challenges for the data industry?
Historically, alternative data was driven by scarcity. High data storage costs led companies to delete aging records, so simply having a dataset with history was a differentiator when talking to data buyers.
However, the landscape has shifted and as the cloud has made data storage and sharing inexpensive and easy, a dataset with history is no longer enough of a differentiator. That said, a lot of companies continue to operate under the assumptions of the bygone era, resulting in a deluge of raw, messy datasets in the market that are difficult and time-consuming to evaluate and use.
This misalignment between data owner and data consumer is the biggest impediment for the data industry. A dataset that is clean, structured, enriched, normalized, and validated against real-world ground truth bridges the gap between data owners and data buyers. However, the expertise and tools needed to perform these steps is in short supply.
Looking ahead, what trends and innovations in the data industry are you most excited about?
I think about the current state of the data industry as version 1.0. A lot of the analysis that is being done is still surface level, with the primary focus on better understanding competitive landscapes or better predictions of upcoming quarterly financial reports.
As the space matures further, I believe we will see movement towards richer insights that offer even deeper understanding of what is happening in the world. Predicting top-line metrics will always have value, but leveraging alternative data to drive insight into nuanced metrics like customer composition, customer retention rates, lifetime value, and brand affinity excites me. These are typically labeled “thesis” oriented insights, but if captured and built into a workflow can deliver far greater value to an end consumer.
Another path to richer insight can come from commingling disparate datasets. Can we weave geolocation data with credit card data to understand the revenue that a store generates for every minute a customer shops? How about Smart TV data with e-commerce receipts to better measure the efficacy of advertising campaigns? I can’t wait for insights like these to become commonplace!
For what datasets do you currently see the most demand from investment managers?
Credit card transaction data remains the gold standard for investors largely because it has been around for such a long time and has embedded itself well in the investment process, but the combination of data proliferation and COVID rendering a lot of transactional data unusable opened the door for historically underrepresented data types like supply chain data and healthcare data. Additionally, as macro trends result in more of our lives occurring online, clickstream datasets are in high demand, though they can be quite difficult to use.
What are the key problems data users face getting data operationally ready?
Similar to the biggest challenges to the data industry, data consumers are often asked to navigate ingestion, QA, and research of raw, messy, and unstructured datasets within a tight trial period window. This means that there’s an additional time and resource investment made in a dataset before a commercial decision is even made, not to mention that incremental investment required to ensure that a dataset, once purchased, is fully integrated into a broader ingestion pipeline.
Aside from a few of the largest funds, the investment industry is still new to these steps, which causes a lot of data to go untrialed and results in missed opportunities for datasets that would otherwise be coveted by investors.
How do you quantify the value of a dataset?
At FirstParty, we have developed the proprietary ANTE Scorecard to determine a dataset’s commercial value. It identifies (A)ccuracy, (N)ovelty, (T)imeliness, and (E)ase of use as the four pillars of value for any dataset, and it scores a data asset against datasets in-market to identify relative outperformance.
In order for a data valuation to be useful, all of these factors must be considered. For example, an accurate dataset that releases with high latency to real-world events might suffer in value relative to a less accurate dataset that is easy to use and has low latency. ANTE takes many scenarios such as this into consideration when determining a dataset’s value, but also (with the proper steps to enrich) its overall potential.
What is your view on alternative data?
The influx of data into any industry is generally accompanied by a degree of initial distrust followed by massive leaps forward in efficiency and performance. Sports offers such a good example – in baseball and basketball, established ways of running a team were upended as new information became available and widely disseminated.
We’ve seen alternative data impact the hedge fund space and drive performance over the past 10-15 years. I think the coming years are going to see more traction across PE and VC firms, as well as corporate and professional services organizations.
Additionally, with third-party cookies scheduled to sunset in 2024, there exists the near-certainty of an information vacuum that will likely be filled by new, emerging alternative datasets that allow advertising dollars to remain targeted and effective.
What is your view on how technological advances such as AI and ML will impact the data and investment management industry?
I tend to be one of the people who thinks about machine learning as a tool rather than a threat to replace. We’re already seeing with large language models how information acquisition becomes a lot less important than information interpretation, and I believe these trends will continue.
As it currently stands, these models are really good at predicting based on the corpus of knowledge they’ve been trained on, but on the investing side, predicting and reacting to paradigm shifts has been a major differentiating factor throughout history, and this is exactly where a lot of traditional ML solutions fall short.
Given all this, I believe the biggest impact that ML will have on investing comes in the form of having more information and more tools available for the investor to utilize in the process – making markets more efficient – rather than replacing the investor altogether.
Disclaimer
SigTech is not responsible for, and expressly disclaims all liability for, damages of any kind arising out of use, reference to, or reliance on such information. While the speaker makes every effort to present accurate and reliable information, SigTech does not endorse, approve, or certify such information, nor does it guarantee the accuracy, completeness, efficacy, timeliness, or correct sequencing of such information. All presentations represent the opinions of the speaker and do not represent the position or the opinion of SigTech or its affiliates.