In the latest issue of SigView, we are thrilled to gain insights from John Lukovich, a portfolio manager at the $73.3 billion Canadian Investment Management Corporation of Ontario (IMCO). John is an esteemed expert in the field of factor investing and he is a key contributor to a multi-billion-dollar systematic equity mandate. Within this interview, he generously shares his perspectives on various topics, including the ongoing AI revolution, his fundamental principles for constructing quant models, and a glimpse into the priorities currently topping his research agenda.
We trust you will find the conversation insightful.
The views expressed in this interview are John Lukovich’s and do not reflect those of IMCO or its affiliates.
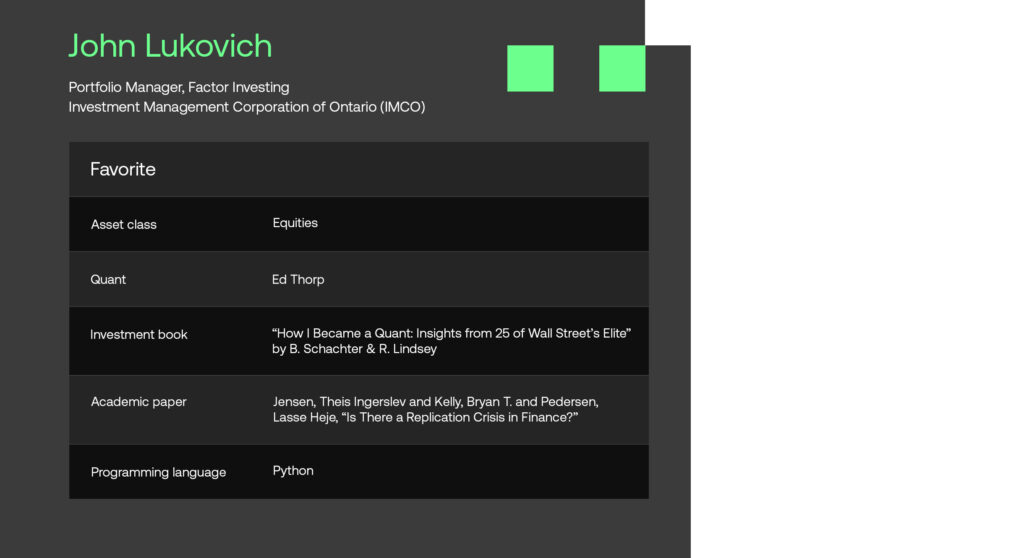
Tell us about your background as a quant.
My first work experience was an internship at Nortel during the Global Financial Crisis. It was during this period that I was introduced to the field of financial engineering, and I immediately fell in love with the idea of applying engineering principles to the financial markets. This made me adjust what I thought my career path was going to look like and I landed at a boutique managed futures shop. My first task was to build a trade reconciliation software for futures trading. This proved to be an invaluable experience as I learned in great detail how futures markets work in practice, but my main interests remained in quant investing. Within a couple years, I was fortunate enough to get the opportunity to work on developing alternative risk premia strategies to enhance diversification of the firm’s core trend strategy.
Following a merger with a quant equity firm, I split my time between researching factor strategies for US and Chinese stocks, and co-running the day-to-day portfolio management activities of the firm’s managed futures strategy. It was a very stimulating environment where I was part of developing and launching multiple investment products, including equity factor, systematic macro, multi-strategy and all-weather strategies for a diverse set of markets such as Canada, China, Korea, and the US.
Today I am at IMCO, where I initially focused on designing a systematic macro portfolio that blended ideas from managed futures, risk parity and alternative risk premia strategies. Currently, my primary focus revolves around developing and running a range of equity factor strategies.
Tell us about IMCO
The Investment Management Corporation of Ontario (IMCO) manages $73.3 billion of assets on behalf of our clients. Designed exclusively to drive better investment outcomes for Ontario’s broader public sector, IMCO operates under an independent, not-for-profit, cost recovery structure. We provide leading investment management services, including portfolio construction advice, better access to a diverse range of asset classes and sophisticated risk management capabilities. As one of Canada’s largest institutional investors, we invest around the world and execute large transactions efficiently. Our scale gives clients access to a well-diversified global portfolio, including sought-after private and alternative asset classes.
What investment strategies do you manage?
My main responsibility at IMCO is running a long-only global equity strategy benchmarked to a globally diversified passive index. Me and the team apply a diversified and balanced approach to factor investing, focusing on factors such as value, momentum, quality, etc. From a risk perspective, the strategy is run on a sector and country neutral basis, and the portfolio’s beta is kept within a tight bound relative to the benchmark. This is done to minimize unintended risks and thus to focus only on harnessing specific risk premia and market anomalies. Medium-term, the strategy targets a modest tracking error.
Moreover, I am actively engaged in the development of a customized strategic factor index with a third-party index provider. This index is subsequently implemented by an external manager, with whom we have a very close working relationship with.
What are your core principles when building quant models as well as creating risk factor portfolios?
My core principles revolve around robustness and diversification. Ideologically, I view investment professionals as a group of economic agents with slightly varying perspectives on maximizing utility — whether from a risk or alpha perspective. My approach towards robustness and diversification is to try to encapsulate the variation in opinions of these investment professionals at key decision points during the investment process. The framework segments the end-to-end investment process into a series of decisions, such as converting investment data into features, translating features into signals, evaluating signal significance based on risk attributes, understanding where signals are in their respective cycles (e.g. price, macro, valuations), and how to convert signals into portfolio weights. I try to identify variations in decisions that investors may take at each step, which in aggregate creates a robust and diversified approach to factor investing.
I liken this process to the binary tree concept of option pricing, but instead of manipulating the path and value of asset prices, I manipulate the path and value of market information. To take the analogy further, instead of looking at basic univariate functions that represent how much an asset can increase or decrease at each layer (time step), with corresponding up/down probability, I look at univariate functions that represent a series of investment decisions on how to interpret the market data at the previous node/layer, and assign each decision an equal probability. As such, each node represents a piece of data, in raw or transformed form, and each node has multiple branches representing a set of univariate functions that corresponds to potential ‘decisions’ that investors can make based on their interpretation of the data. As you layer on more decisions to each piece of transformed data, you create a data structure that resembles a tree, or a network. The final ‘leaves’ of this network becomes a set of simplified quant portfolios sourced from a single, or combination of, investment data. Combining the leaves from different trees creates an extensive set of possible portfolios. Relating this back to factor investing, the leaves, each of which represents a sequence of investment decisions culminating into a possible portfolio, covers much of the investable factor landscape. Further, as many of the resulting portfolios have similar characteristics, they form themes or clusters, culminating in factor portfolios when grouped and averaged. Constructing factors this way increases robustness, providing a purer representation as it encompasses varied measurements of each factor during the process of mapping data to a final portfolio.
Lastly, having such a framework also allows for filtering out poor sequences of decisions, or putting less weight on them in the final portfolio construction. Each of these ‘filtrations’ implicitly assumes a probability measure on the subset of portfolio decisions, which is yet another way to create diversification and robustness into the portfolio management process.
What is currently on top of your research agenda?
I am currently focusing on how to minimize effects from unintended risks in the equity factor portfolios that the team manages. The overarching idea is to ‘purify’ the exposure of specific factors and thus minimizing any effects that other risks may have. We ask ourselves, what are the additional risks we should monitor, and how can we best neutralize them from our core portfolio drivers? In an ideal scenario, I’d prefer a risk contribution from systematic risk factors that we control and purify, while minimizing the risk contribution from unintended sector, region, beta and even macro risks.
In addition, I’m exploring ideas of how to adjust the number of stocks in the investment universe to maximize the likelihood of achieving the sufficient breadth that is often required to achieve successful outcomes in long-only factor investing. To explain the logic of this idea, we start with the premise that a core structural problem in long-only equity factor investing is that it doesn’t allow you to perfectly express your view/signal into an active weight (imperfect transformation coefficient). This constraint stems from the fact that the maximum extent to which you can adopt a ‘short’ position is limited by the size of the stock in the benchmark. The main unintended effects of this structural problem are twofold: firstly, your long signals are more important than your short signals, and secondly, you often resort to leveraging larger cap names as funding sources for your smaller cap names. Both of these outcomes result in more trading of your larger cap names, and worse over, you often end up having unintended exposures in these names. The effects of this are magnified when the performance of broader benchmarks are driven by large-caps. Returning to the context of the research involving adjusting the number of stocks in my investment universe, the objective is to identify which stocks to keep at benchmark weight. This is done to mitigate some of the unintended effects of the imperfect transformation coefficient when doing long-only equity factor investing. This challenge is particularly pronounced during periods of small market breadth, such as the current environment.
What is your perspective on technological advancements such as AI and machine learning, as well as alternative data? Are we currently experiencing a paradigm shift?
While I don’t claim to be an expert in AI and machine learning, as a quant, I find the recent advancements in these fields undeniably exciting. However, it’s important to note that we’re still in the early stages, and I believe that a true paradigm shift in alpha generation is yet to materialize. Predecessors to current models, such as neural networks, have existed for quite some time, without having a major impact on our industry. Many older generation tree-based models still provide the best results.
Nonetheless, it’s reasonable to conclude that these advancements are significantly impacting productivity. As an example, the emergence of LLMs (large language models) has notably improved NLP (natural language processing) analysis. Additionally, tools like LangChain make it easier to streamline the transformation of a series of prompts into a cohesive framework, thus simplifying the deployment of certain workflows.
Lastly, while I won’t go as far as calling it a paradigm shift, I foresee shrinking barriers to entry of applying modern quant analytics towards investment decision making as AI tooling continues to improve. This will naturally result in the blurring of the line between fundamental and quant investing as the toolset overlap increases. However, good analytics requires good data, so the real kicker towards this ‘paradigm shift’ has been the maturing of the data engineering profession in finance. Easily accessible, intuitive, and interpretive ML tooling mixed with high-quality data will continue to make markets more efficient and will continue to spur financial innovation. This makes working in the financial industry an exciting place to be.
What are the key challenges you face in your day-to-day operations developing and maintaining quant models?
One of the key challenges I face is establishing a framework that effectively evaluates the ongoing relevance of market data in the price discovery process. While assessing the historical performance of a strategy based on past data is relatively straightforward, predicting its future applicability presents a greater challenge. A case in point is the evolution of traditional low-frequency earnings data as a reliable indicator for future equity performance. Recent advancements in data availability and big data workflows allow you to leverage proxy variables with higher granularity than corporate earnings, fundamentally altering their effectiveness on a permanent basis. This prompts the question of how many investors only rely on corporate earnings to make decisions, as opposed to those who rely on more timely estimates, and how this dynamic influences equity prices. The challenge lies in knowing when to stop using data that has become encapsulated by a quicker or more accurate source of information.
Operationally, ensuring data is of high quality is fundamental to quant investing. The old adage of ‘garbage-in garbage-out’ is probably the only thing that quant investors can collectively 100% agree on. This naturally applies to the process of extracting raw data, handling missing data, and manipulating the final production data, which is essential for storage and utilization in the investment models. Developing automated integrity checks for this process is always challenging, as issues frequently arise that necessitates manual intervention. A good example is checking corporate fundamentals. Various vendors adopt different assumptions about how to process certain expense items. Consequently, cross-checking across vendors to ensure consistent processing of the final stored data can prove time-consuming. Once we have the data ready, we work closely with the IT team to integrate some model-running workflows into our cloud infrastructure. During this process, it can be challenging to properly migrate any new package dependencies into our cloud production environment due to dependency conflicts. This is because the cloud infrastructure already has a series of required packages that are independent of our development environment, so we often end up relying on brute force iteration until our tests pass.
Moreover, as an equity manager, I also grapple with the complexities of ESG data. Firstly, it involves understanding the relevance of individual ESG variables. Secondly, there’s the task of reconciling the disparities in data provided by various ESG data providers. Given that companies know that their ESG scores matter to numerous institutional investors, effectively identifying greenwashing becomes crucial.
More broadly, keeping abreast of broad market themes that come up from time-to-time, deciding on how to best measure your portfolio’s sensitivity to such themes, and also on whether or not to take an active bet on them.
Where do you see the most promising opportunities to generate alpha in the current market environment?
I believe that we will continue to experience a market driven by macro themes. Consequently, integrating macro insights into the portfolio construction process will be key. I anticipate that neutralizing systematic factors to such macro themes to minimize unintended macro exposures is a better idea in the near term. However, as key macro themes become entrenched into the economy – such as the ‘higher for longer’ scenario or subdued global growth – adding strategic tilts within an equity factor portfolio may be a good idea. To effectively execute this, a robust macro risk model that assesses single stock sensitivities to such characteristics is important.
In addition, I believe that incorporating insights from the credit market to enhance equity factors is important, especially from a risk management perspective. Typically, the initial signs of trouble emerge within credit, and equity managers are almost always caught off guard. Understanding concepts like liquidity for new issues and default probabilities is crucial. Furthermore, I’m excited by the chatter and potential expansion of applying factor investing to credit. I believe this will uncover new avenues of cross-pollination between the credit and equity investment landscapes.
I also believe in exploring new sources of data and to utilize existing data in new ways to improve forecasting capabilities. Returning to my previous point about company earnings, nowcasting is a field I believe will enable managers to gain, or perhaps rather keep their edge. It boils down to what data investors use to make investment decisions, and how early in the information stream data is accessed to stay ahead of the curve when measuring risk premia.
What resources do you recommend for staying informed about the latest advancements in quantitative finance?
On LinkedIn, I actively follow a handful of mid-career quant professionals who frequently share insightful perspectives on tackling concrete implementation challenges. Two that I enjoy reading insights from are Vivek Viswanathan and Christina Qi. For broader insights into macro and asset allocation, I find John Normand’s posts particularly interesting. To stay up-to-date with interesting AI/ML trends, I follow Allie Miller and Bojan Tunguz.
In terms of podcasts, I recommend ‘Flirting with Models’ by Corey Hoffstein. It goes into some interesting details for designing systematic trading strategies. Another excellent podcast is ‘QuantSpeak’, presented by the CQF Institute, offering similar content but with a stronger focus on research topics from an academic perspective. ‘Data Skeptic’ by Kyle Polich is a great resource for learning about AI/ML tools, and I enjoy the interviews conducted by Barry Ritholtz on the ‘Masters in Business’ podcast.
Lastly, I recommend research from specialized hubs. Examples include academic sources like EDHEC, as well as industry-focused firms such as ADIA Lab and AQR.
Disclaimer
Sig Technologies Limited (SigTech) is not responsible for, and expressly disclaims all liability for, damages of any kind arising out of use, reference to, or reliance on such information. While the speaker makes every effort to present accurate and reliable information, SigTech does not endorse, approve, or certify such information, nor does it guarantee the accuracy, completeness, efficacy, timeliness, or correct sequencing of such information. All presentations represent the opinions of the speaker and do not represent the position or the opinion of SigTech or its affiliates.